AI’s Impact on Inequality
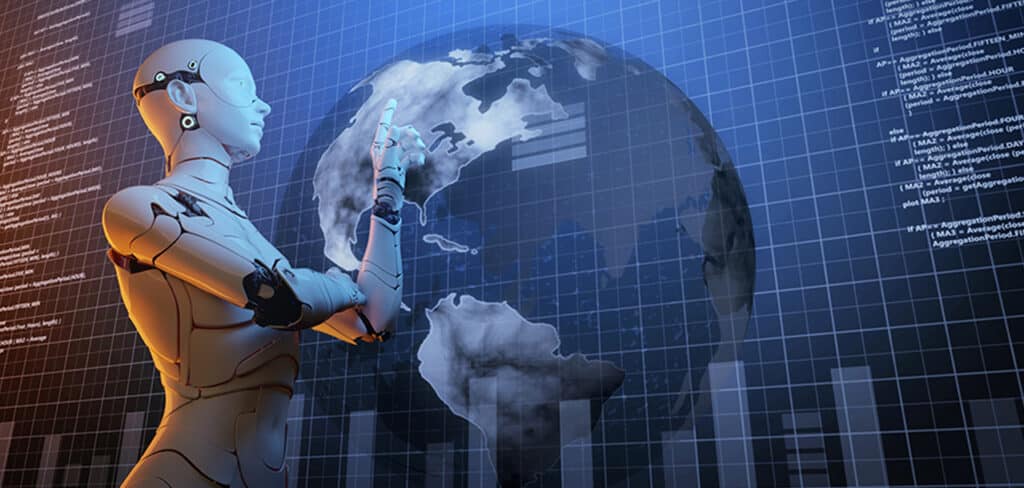
Introduction
Artificial intelligence is transforming our societies at breakneck speed. From healthcare to finance, recruitment to energy, it promises revolutionary change. But behind the glossy headlines lies a harsher truth: AI is not an equalising force. Rather, it often amplifies the very inequalities it could be used to fix. What emerges is a dynamic Bhaskar Chakravorti, the Dean of Global Business at The Fletcher School at Tufts University and founding Executive Director of Fletcher’s Institute for Business in the Global Context, terms “artificial inequality,” where AI “concentrates socioeconomic opportunities and outcomes within narrow societal segments while depriving others” [1].
Through six intersecting divides –– data, income, usage, geography, industry, and energy –– this article explores AI’s impact on inequality, examining how each divide manifests and what, if anything, companies can do to stop AI from becoming a 21st-century tool of exclusion.
The data divide
Data is the lifeblood of AI, but it’s also where inequality begins. While the algorithms themselves may be neutral, the datasets they are trained on often reflect deep societal biases. Swati Tyagi, a seasoned AI/ML professional who has made significant contributions to gender equity in AI and explainable credit scoring, notes that “many AI systems demonstrate significant gender bias, particularly disadvantaging women and women of color,” a result of overrepresenting white male experiences in data and design [2].
Chakravorti reinforces this, citing examples like mortgage algorithms rejecting Black applicants at rates 150% higher than white counterparts in Chicago, and 200% higher for Latino applicants in Waco, Texas [3]. In healthcare, biased training data has led to AI diagnostic tools systematically underdiagnosing women and patients of colour.
This data divide creates a feedback loop: biased algorithms make discriminatory decisions, which produce further biased data, worsening outcomes over time. Companies have a responsibility to address this, not only through de-biasing datasets but also by collaborating with external institutions like the Algorithmic Justice League, which provides training tools and advocacy for awareness-building, and IBM’s AI Fairness 360 Toolkit [4].
The income divide
One of the most visible ways AI drives inequality is by increasing income gaps. High-skilled workers in AI-complementary roles see productivity and wage boosts, while others — especially in routinised jobs — face obsolescence. The IMF estimates nearly 40% of jobs worldwide will be affected by AI, with even conservative projections from the likes of Nobel laureate Daron Acemoglu predicting 5% of tasks will be replaced within a decade [5].
Joseph Stiglitz, a renowned economist and recipient of the Nobel Prize in Economics in 2001, is blunt about the consequences: “the net effect will be to reduce the demand for labour, increase inequality, [and] increase unemployment” [6]. This is especially worrying in low-skilled sectors and emerging economies. Even more troubling, few governments have plans to cushion the blow. The World Economic Forum found only 22% of countries have policies to address AI’s labour disruptions [7].
Yet businesses do have tools to help. Investing in reskilling programmes, partnering with initiatives that offer hands-on experience in AI tools to a wide cross-section of users like AI4ALL or Charity Excellence, or developing inclusive hiring AI are practical steps. As Chakravorti points out, many smaller firms can use AI analytics to reduce costs and compete with larger rivals, which could help level the income field if rolled out inclusively [8].
The usage divide
Even as AI spreads, its usage remains uneven — both across regions and among different socioeconomic groups. In the US, for example, those with higher education and income are far more likely to use AI tools and trust their results [9]. The roots of this usage divide are technological, but also psychological: mistrust in AI systems — especially those seen as opaque or unreliable — keeps many away.
The implications are clear. “People who use [AI] will be better positioned to navigate the coming change,” Chakravorti writes [10]. Tools exist to build trust — diagnostic systems with expert validation, interpretability features, and feedback loops — but companies must prioritise them. Without trustworthy AI, usage gaps will grow, worsening digital exclusion.
The market incentives are real. Research shows that customers are twice as likely to use AI tools they trust, and workers are 2.5 times more likely to use employer AI systems if they believe in their reliability [11]. Those who invest in trustworthy design,like Salesforce with its “humans at the helm” pledge, are already reaping reputational benefits.
The global divide
Just as AI polarises within countries, it also exacerbates gaps between them. High-income countries stand to benefit the most, with 60% of jobs exposed to AI (and potentially productivity-enhancing) compared to just 26% in low-income nations [12].
Clinton Vince, head of Dentons’ US Energy Practice, warns that “the digital divide between developed and developing nations is very, very real,” pointing to Sub-Saharan Africa’s electrification struggles and AI’s potential to help — if access can be improved [13]. Yet current US policies restricting access to advanced chips could widen these divides further.
One solution lies in open-source AI. Companies like DeepSeek in China have shown that open-weight models can rival proprietary ones at a fraction of the cost and resource demand [14]. When AI is democratised, local innovations can thrive — like Plantix, an app helping smallholder farmers identify pests. Global AI doesn’t have to mean global monopoly.
Ireland presents a compelling case study in navigating the global AI divide. Despite its small size, the country has emerged as a hub for European AI development, bolstered by a highly educated workforce and favourable tax environment. Yet questions remain about whether this growth is broad-based or disproportionately benefits multinational tech firms clustered around Dublin. Without investment in regional infrastructure and digital upskilling beyond the capital, Ireland risks creating a two-tier tech economy — one concentrated in elite urban sectors, and another left behind in rural areas. The challenge for policymakers is to ensure AI supports inclusive innovation rather than deepening existing regional disparities.
The industry divide
Perhaps nowhere is inequality more stark than in the concentration of AI power. Tech giants dominate the value chain, reinforced by exclusive deals and hardware monopolies. Meta relies on Amazon’s cloud, and most AI firms depend on Nvidia’s chips. Chakravorti notes this “locks out new entrants” and skews investments to commercially safe bets, ignoring social impact [15].
Stiglitz warns this dynamic leads to “unchecked market power” and “entrenched monopoly,” where economic concentration begets political influence [16]. Nvidia’s $2.7 trillion valuation and 88% GPU market share aren’t signs of healthy competition — they’re signals of consolidation.
To mitigate this, companies can adopt “edge AI” or small models tailored to specific use cases. Open-source alternatives, including from European firms like Mistral, also show promise. Tools like the Digital Public Goods Alliance,a multi-stakeholder initiative, promote open-source software, open data, open AI models, and open content collections that adhere to privacy standards. If more firms embrace diverse sourcing and modular openness, the industry could begin to rebalance.
The energy divide
AI’s energy appetite is another major contributor to inequality. Data centre energy use is set to rise by 35% by 2026, with water and electricity demands soaring [17]. While large firms can absorb these costs, others — particularly in the Global South — cannot. And many firms risk missing their net-zero targets due to AI’s emissions.
Yet innovation offers hope. Google’s DeepMind has cut cooling energy by 40%, and Nvidia’s newer chips use 25 times less energy than prior models. DeepSeek has shown how smart model design, like using fewer decimal places or “mixture of experts” techniques, can dramatically reduce energy needs [18].
Companies can also build greener AI by locating data centres in energy-efficient regions and using tools like photonic accelerators or 3D chips. Meanwhile, initiatives like the Cornell AI for Sustainability programme are pushing best practice on how to manage AI’s energy use, as well as how AI can help us use energy more efficiently. As Chakravorti argues, “efficiencies contribute to overall cost and resource efficiencies and enhance competitiveness” [19].
The gender divide
While often overlapping with the data divide, gender bias deserves distinct attention. AI tools reflect the unequal world they are trained on. As Swati Tyagi explains, algorithms increasingly drive healthcare, employment, and finance — but too often, they do so in ways that “systematically exclude women” [20].
In hiring, AI tools penalise women for career breaks (the “mom penalty”). In finance, credit algorithms reject women at higher rates than equally qualified men. In healthcare, diagnostic tools underperform on women, especially women of colour [21]. These aren’t isolated glitches — they’re systemic outcomes baked into the data and design.
Fixing this requires more than technical audits. Diverse development teams, sector-specific fairness rules, and mandatory transparency in AI decision-making must become the norm. If left unaddressed, AI risks rolling back decades of gender equality gains.
Monopoly, market power, and the 1%
Ultimately, the question is not just what AI can do, but who it serves. Joseph Stiglitz sees in AI an accelerant of monopoly capitalism, one that feeds a feedback loop of wealth and influence: “When market power becomes highly concentrated… that economic influence translates into political power” [22].
Already, the top 10% of US households control 67% of wealth; the top 0.1%, which includes major AI stakeholders, have seen their share of wealth grow from 8.6% in 1990 to 13.8% today [23]. The richest five tech billionaires alone have added $1 trillion to their fortunes in the last nine months.
This concentration undermines Adam Smith’s ideal of self-interest leading to collective good. Stiglitz argues we are instead witnessing a system where the gains of AI accrue to the few, while the costs — lost jobs, degraded trust, ecological strain — are socialised. Only with regulation, competition policy, and aligned incentives can this tide be turned.
AI’s impact on inequality
AI’s future is not set in stone. As Chakravorti writes, “AI acceleration does not have to translate into a more fragile, divided world” [24]. But it will, unless proactive measures are taken. Companies can act: by investing in fair data, trustworthy design, inclusive access, open ecosystems, and sustainable operations. Governments must also play their part, through regulation, reskilling programmes, and digital infrastructure.
Left to its own devices, AI will reflect and reinforce our worst inequalities. But guided wisely, it still holds the potential to help us build a fairer world.
More on AI
The Ethical Minefield of Artificial Intelligence
The EU AI Act: What you Need to Know
AI – A doomsday scenario with Roman Yampolskiy – Podcast